What Role Does AI Play in Securing IoT Networks?
As the digital world continues to expand, organizations must view AI not just as a tool but as a strategic cornerstone in their cybersecurity efforts. Those who adopt AI early and wisely will stand stronger against the evolving landscape of cyber threats.
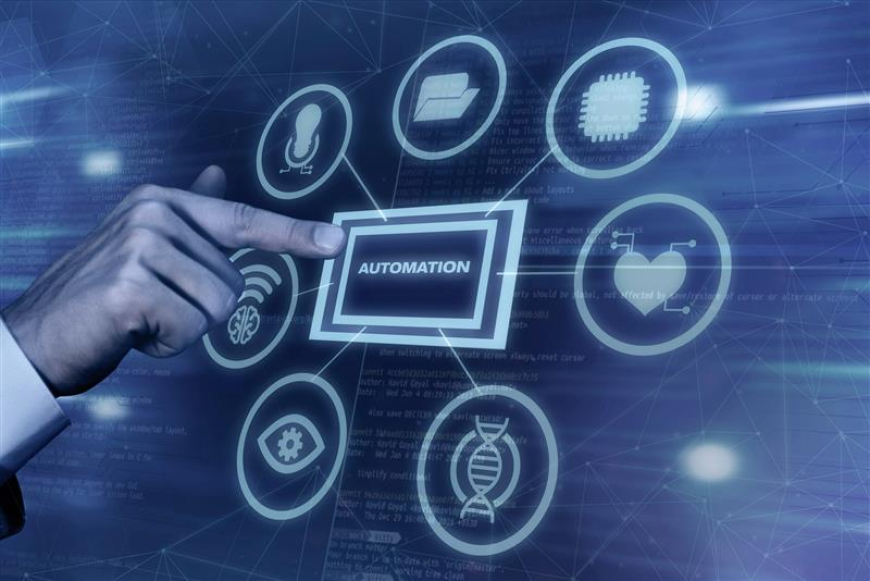
The Internet of Things (IoT) has rapidly expanded, connecting billions of devices globally — from household appliances and wearable tech to industrial sensors and autonomous vehicles. While this interconnectivity offers convenience and innovation, it also brings significant cybersecurity risks. Traditional security frameworks struggle to address the massive, dynamic, and resource-constrained environments IoT creates.
This is where Artificial Intelligence (AI) steps in. AI offers scalable, intelligent, and automated solutions to secure IoT networks, ensuring resilience against both known and emerging cyber threats. In today's highly connected world, businesses often rely on customized AI solutions, sometimes collaborating with an expert ai software development company in nyc to meet their evolving IoT security needs.
Understanding the Unique Challenges of IoT Security
Scale and Diversity
IoT ecosystems often consist of millions of devices, each varying in design, function, and security capabilities. This diversity makes it difficult to apply a one-size-fits-all security solution.
Limited Computational Resources
Most IoT devices have minimal processing power and memory, making them unsuitable for traditional security measures like robust encryption or constant antivirus scanning.
Continuous Connectivity
IoT devices operate 24/7, often over open networks like Wi-Fi or mobile connections, making them vulnerable to persistent threats such as DDoS attacks and ransomware.
Physical Exposure
Many IoT devices are deployed in physically accessible areas — homes, offices, factories — making them susceptible to tampering or theft, leading to further vulnerabilities.
Why Traditional Security Solutions Are Insufficient
Traditional network security relies on perimeter-based defenses: firewalls, VPNs, and centralized intrusion detection systems. However, in an IoT environment:
-
Devices constantly move across networks.
-
Attack surfaces are highly distributed.
-
Firmware updates are often delayed or skipped.
This decentralization demands a more adaptive, self-learning security model — precisely what AI offers.
How AI Strengthens IoT Security
Real-Time Threat Detection
AI systems can continuously monitor IoT devices, analyzing large volumes of data to identify suspicious patterns. Unlike rule-based systems that recognize only known threats, AI models can detect previously unseen attacks through behavioral analysis.
Predictive Analytics
Through machine learning, AI can forecast potential vulnerabilities based on historical data, environmental changes, and device behavior. Predictive analytics allows enterprises to implement preemptive security measures rather than reacting to attacks after they occur.
Autonomous Response
Upon detecting anomalies, AI-powered systems can autonomously:
-
Quarantine infected devices.
-
Modify firewall settings.
-
Initiate firmware patches.
This swift, automated response minimizes damage and downtime, crucial for mission-critical environments like healthcare and manufacturing.
Adaptive Learning
IoT ecosystems evolve rapidly, with devices added or modified frequently. AI solutions can adapt in real time, retraining their models to accommodate changes without manual intervention.
Applications of AI in IoT Security Across Industries
Healthcare
In hospitals, where IoT devices monitor patient vitals, AI helps detect if devices are compromised. Immediate responses protect sensitive patient data and ensure life-saving equipment remains functional.
Smart Cities
Urban infrastructure—traffic systems, surveillance cameras, public utilities—relies heavily on IoT. AI aids in proactively identifying vulnerabilities, ensuring public safety and operational efficiency.
Manufacturing and Industrial IoT (IIoT)
Factories deploy thousands of sensors and smart machines. AI monitors data flows for signs of cyberattacks that could halt production lines or cause equipment failures.
Automotive
Connected vehicles depend on IoT for navigation, communication, and entertainment. AI secures vehicular networks, safeguarding against threats that could endanger lives.
Core AI Technologies Used in Securing IoT Networks
Machine Learning (ML)
Machine learning algorithms train on historical and real-time IoT data, learning to distinguish between normal operations and anomalies that may indicate attacks.
Deep Learning
Through neural networks, deep learning models can recognize intricate threat patterns across multiple devices and layers of a network, enabling highly accurate anomaly detection.
Natural Language Processing (NLP)
In devices featuring voice or text interfaces, NLP-based AI monitors and filters suspicious commands that could signify an attempted exploit.
Reinforcement Learning
Reinforcement learning enables AI models to improve through trial and error, refining their responses to threats over time for maximum effectiveness.
Computer Vision
Security cameras and other visual IoT devices benefit from AI-driven computer vision, detecting unauthorized access, vandalism, or environmental hazards in real-time.
The integration of these sophisticated AI capabilities is often enhanced by partnerships with a specialized ai based chatbot development company, especially when natural human-device interactions are involved.
Key Advantages of Using AI in IoT Security
Scalability
AI systems easily scale to monitor millions of devices simultaneously, a necessity given the exponential growth of IoT deployments.
Speed and Efficiency
AI processes vast datasets far quicker than human operators, enabling faster detection and response to threats.
Reduced Operational Costs
Though initial implementation might seem costly, AI-driven security minimizes the need for large manual cybersecurity teams and reduces recovery expenses after breaches.
Improved Accuracy
Self-learning AI models continue to refine their understanding of normal versus abnormal behavior, resulting in fewer false positives and missed threats.
Challenges and Risks of AI in IoT Security
Data Privacy Concerns
AI models require extensive datasets to function optimally. Improper handling of this data could result in serious privacy violations, emphasizing the need for strong data governance policies.
Complexity of Deployment
Integrating AI security solutions into existing IoT frameworks demands careful planning, high technical expertise, and considerable customization. Many enterprises choose to outsource asp.net development to incorporate AI modules seamlessly into their platforms and applications, accelerating time to market.
Adversarial AI Attacks
AI itself can become a target. Malicious actors can use adversarial machine learning techniques to deceive AI models, making threat detection harder.
Transparency and Accountability
Black-box AI models make it difficult to explain why certain actions were taken, which can create trust issues, especially in highly regulated industries.
The Future: How AI Will Further Revolutionize IoT Security
Edge AI and Decentralized Processing
Processing data closer to the source (on IoT devices themselves) through edge AI reduces latency and enhances security by minimizing the amount of sensitive data transmitted over networks.
Federated Learning for Privacy
Federated learning enables devices to learn collaboratively without sharing raw data, significantly enhancing user privacy and security.
AI-Driven Self-Healing Networks
Future AI systems will not only detect and neutralize threats but also automatically repair network vulnerabilities, creating resilient, self-healing IoT ecosystems.
Explainable AI (XAI)
Explainable AI will offer greater transparency by clarifying how and why security decisions are made, building greater trust among users and regulators.
Conclusion
The explosive growth of IoT devices has fundamentally reshaped modern life, but it has also introduced unparalleled security challenges. Traditional methods are no longer adequate for the scale, complexity, and speed at which threats evolve in IoT ecosystems.
Artificial Intelligence brings a transformative approach to IoT security — offering real-time threat detection, predictive insights, autonomous responses, and continuous adaptation. While challenges such as data privacy and deployment complexity remain, advancements in edge computing, federated learning, and explainable AI are paving the way toward more secure, resilient, and transparent IoT networks.
As the digital world continues to expand, organizations must view AI not just as a tool but as a strategic cornerstone in their cybersecurity efforts. Those who adopt AI early and wisely will stand stronger against the evolving landscape of cyber threats.