What Is Predictive Analytics And How Does It Work?
Predictive Analytics is essential for efficient decision-making in a fast-paced market.
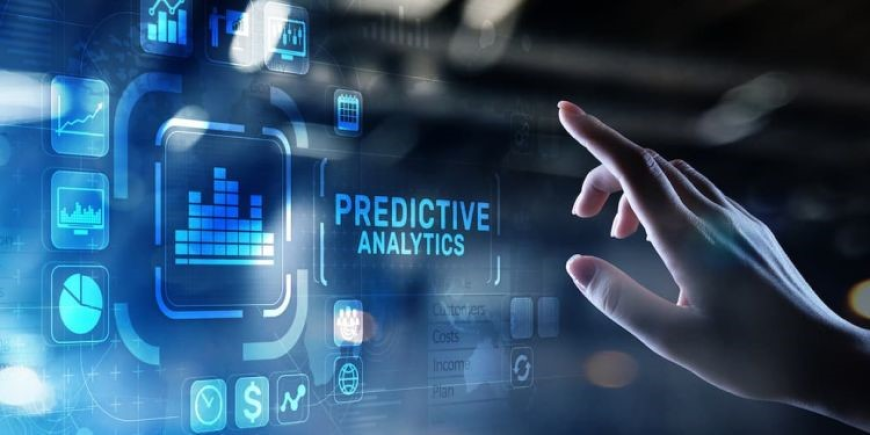
Introduction
Predictive Analytics is a growing technology in 2025, mainly used to forecast future events. Historical data, statistical techniques, and machine learning is used to predict future trends. Today, marketing, finance, healthcare, and manufacturing sectors use Predictive Analytics to meet business objectives. This technology facilitates results prediction, which enhances decision-making for organizations. It identifies hidden patterns in large data and transforms raw data into perceptual analysis. Predictive Analytics is essential for efficient decision-making in a fast-paced market. Refer to the Business Analytics Courses in Delhi to learn more about Predictive Analytics.
All About Predictive Analytics
Predictive Analytics is a subfield of Advanced Analytics. As mentioned earlier, it works upon historical data, algorithms, and machine learning to predict future outcomes. By detecting trends and patterns in present data, it rule of thumb for projections about future events or behaviour.
Finance, marketing, healthcare, and manufacturing industries function based on Predictive Analytics today. Using this technology, businesses can reduce risks, fast-track business processes, and make effective data-driven decisions to meet their goals. Data collection, cleaning, model building, and evaluation are the vital components of this technology. Moreover, Predictive Analytics uses regression analysis, neural networks, decision trees, and clustering to predict more accurate outcomes. Python, SAS, R, IBM SPSS, RapidMiner, etc., are commonly used for Predictive Analytics in modern times.
The retail industry uses Predictive Analytics to understand market demand and customize offers for the customer. In healthcare sectors, it helps project patient risk or sickness outbreaks. Thus, with Predictive Analytics, businesses are able to boost their efficiency by understanding upcoming trends. Moreover, it helps companies stay competitive in the market through effective decision-making.
How Does Predictive Analytics Work?
Predictive Analytics predicts future results by means of historical data. The procedure combines model evaluation with machine learning techniques, statistical analysis, and data gathering. It helps companies to trend-spot, behaviour-predict, and act pre-emptively. One can check the Business Analysis Training courses for more information.
1. Data Collection and Preparation
The first stage is compiling information from several databases, consumer transactions, social media, sensors, and logs. Including both organized data (e.g., numbers, dates) and unstructured material (e.g., text, visuals).
Data is cleaned and ready for analysis once it is gathered. This phase encompasses:
- Dealing with missing data
- Removing duplicates
- Normalizing and transforming data
- Feature engineering to generate fresh variables that might enhance predictions
2. Exploratory Data Analysis (EDA)
Through visualizations and statistical summaries, Exploratory Data Analysis (EDA) seeks to grasp the data. Trends, correlations, and anomalies that could affect the predictive model are found by analysts. This helps in choosing the appropriate approaches and variables for modelling.
3. Model Building
In this stage, the cleaned dataset is used to create statistical models or machine learning. Common modelling approaches consist in:
- Regression Analysis: Offers a steady value (e.g., sales forecast) prediction.
- Classification Models: Predict categories (e.g., churn vs. no churn).
- Decision Trees and Random Forests: Tree-like models dealing with complexity in decisions.
- Neural Networks: Mimic the human brain to negotiate nonlinear patterns.
- Clustering Algorithms: Organize data points with related traits.
These models are built on historical data, learning the links between input variables and outcomes.
4. Model Evaluation and Validation
The model's accuracy is tested using new, “unseen” data (test data). Performance is assessed using evaluation measures such as accuracy, recall, F1 score, and RMSE (Root Mean Square Error). Deployment of the model is considered if it performs well; otherwise, it is improved and retrained. Aspiring professionals can join the Business Analyst Training in Gurgaon to learn more about the different steps involved in Predictive Analytics.
5. Deployment and Monitoring
Validated models are sent into live surroundings. It can now forecast real-time data. Checking the model's performance over time guarantees it keeps producing correct results. As data patterns change, models might have to be retaught.
Real-World Applications
- Marketing: Predict which consumers are most likely to respond to campaigns
- Finance: Predict stock values, find fraud, or determine credit risk.
- Healthcare: Predict disease risks, readmissions, or treatment outcomes
- Manufacturing: Predictive maintenance calls for the expectation of equipment failures.
Conclusion
Predictive Analytics is a potent instrument for turning historical data into workable ideas. By following a systematic process of data gathering to model deployment, companies can reduce risks, get a competitive advantage, and improve decision-making based on projected results.